New App Analyzes Tweets to Prevent Food Poisoning Outbreaks
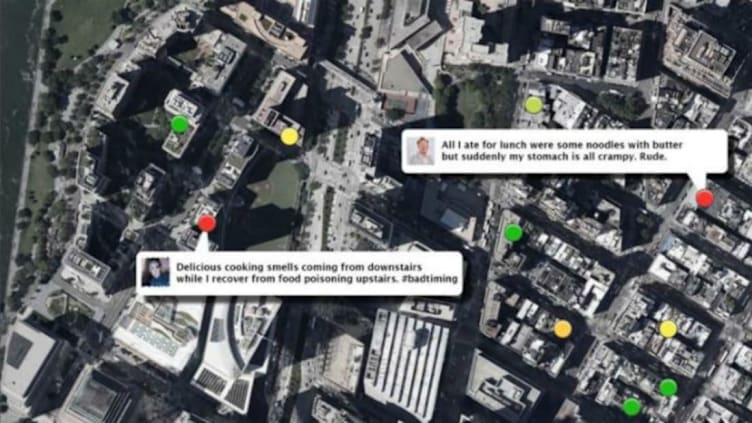
Being sick is awful, but complaining often helps. In the future, your whining may help someone else. Computer scientists have found a way to use tweets complaining about food poisoning to track and prevent outbreaks. The researchers presented their results [PDF] at the annual meeting of the Association for the Advancement of Artificial Intelligence.
Geolocation and public health have a long and storied relationship going back to the 1800s, when physician John Snow noticed a relationship between specific water pumps in London neighborhoods and the number of nearby people who died of cholera. At the time, physicians believed that cholera was called by “bad air.” Snow walked through cholera-stricken neighborhoods, talking to residents and watching where the pump from the water went. With this data, Snow was able to draw a precise map of pump usage, unequivocally proving that the water was to blame. (Unfortunately, it would take several years and many more cholera deaths before his “germ” theory was taken seriously.)
We’ve come a long way since Dr. Snow, but contamination-related outbreaks remain a huge issue. Health departments do what they can with regular restaurant inspections, but they simply can’t be everywhere all of the time. Fortunately, there’s Twitter—and nEmesis.
nEmesis is a cleverly named app (“emesis” is the medical term for puking) with a single purpose: to pinpoint the epicenter of clusters of food poisoning-related tweets. The researchers reviewed thousands of tweets, then compiled a list of the most common terms related to food poisoning.
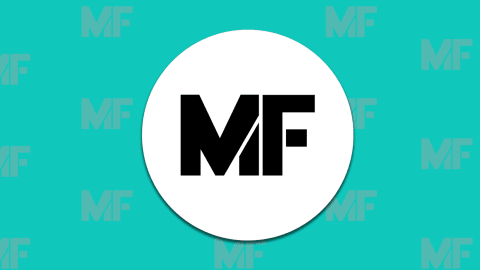
The appearance of any of those terms represents a hit. When enough hits appear in a given geographic area, the nEmesis algorithm can be pretty sure there’s a contaminated kitchen nearby. With enough data, the app can pinpoint outbreaks to a single restaurant.
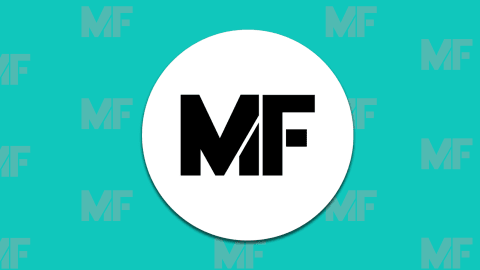
"We don't need to go door to door like John Snow did," nEmesis co-creator Adam Sadilek said in a press statement. "We can use all this data and mine it automatically."
Sadilek and his colleagues decided to test nEmesis in Las Vegas, a city that could fairly be called the buffet capital of the world. They gave the app to one group city health department workers, who used it to prioritize their kitchen inspections. A control group of workers were given an app that provided random suggestions as to which restaurants to inspect.
The researchers collected and analyzed three months’ worth of data from nEmesis and the health inspectors who used it. About 9 percent of the randomized health inspections found citation-worthy violations. The nEmesis-suggested inspections, on the other hand, yielded a 15 percent citation rate. Some of the restaurants involved were given warnings, while others were closed altogether.
Sadilek and his team say nEmesis likely prevented 9000 incidents of food poisoning and 557 hospitalizations.
"Adaptive inspections allow us to focus our limited resources on the restaurants with problems," communicable disease expert Brian Labus said in the press statement. "The sooner we find out about a problem, the sooner we can intervene and keep people from getting sick."
Sadilek noted that food poisoning is just the beginning.
"This happens to be restaurants, but the method can also be used for bedbugs," he said. "Similarly, you can look what people tweet about after they visit their doctor or hospital. We're just beginning to scratch the surface of what's possible."
All images from Adam Sadilek, University of Rochester