Dr. Lydia E. Kavraki: A Woman Making Robots Work
By Kate Erbland
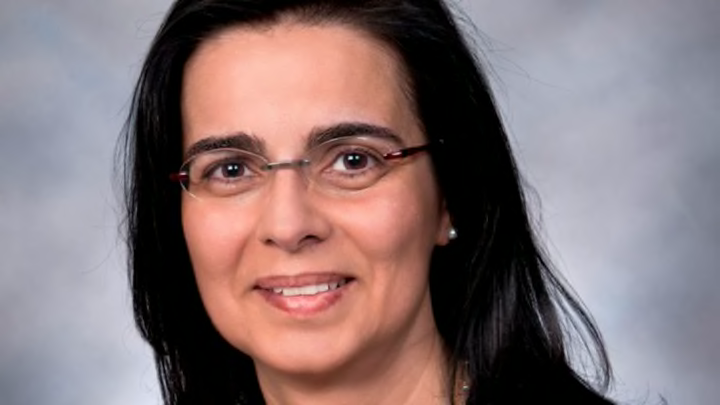
Dr. Lydia E. Kavraki’s list of accomplishments, accolades, and titles is almost too much to process—the computer scientist is currently the Noah Harding Professor of Computer Science and Bioengineering at Rice University, but she also holds an appointment at the Department of Structural and Computational Biology and Molecular Biophysics at the Baylor College of Medicine in Houston, in addition to sitting on a number of advisory boards for various publications, holding fellowships and memberships at a whole mess of associations and institutions, and running her own lab at Rice. It may sound a bit dry, until you realize what Kavraki’s work consists of: She makes robots work. It’s not bad work if you can get it (or, alternately, you’re brilliant enough to do it).
Kavraki’s work is incredibly complex (to put it mildly), but her robotics work essentially boils down to path planning for robots—making sure they have a collision-free path to follow. Her method, the Probabilistic Roadmap Method (PRM), is hailed for providing a paradigm shift across the robotics community, as it utilizes randomizing and sampling-based motion planners to path plan, a simpler technique than had been previously used (one that meant that all applicable path space had to be explored and taken into account). Kavraki also helped write the book on the subject—literally: Her Principles of Robot Motion is the preeminent text on the subject. She also developed the Open Motion Planning Library, part of the Robot Operating System, which is referred to as “the Unix of robotics”—it’s that essential to modern robotic movement. Kavraki’s research is highly applicable across all sorts of robotics, including previously unsolvable problems like how to dock an airspace shuttle to an orbiting space station and “teaching” robots how to tie knots when suturing in a surgical environment.
Kavraki’s expertise also extends to the world of bioinformatics, and her work there applies to the structure and flexibility of molecules, just in case she didn’t have enough to do already.
In 2000, Kavraki won the Association for Computing Machinery (ACM) Grace Murray Hopper Award for her technical contributions, an incredibly special award that only goes to a computer professional who makes a single, significant technical or service contribution at or before age 35. (How special? On five occasions, the award wasn’t given out; their standards are just that high.) She’s also got a Sloan Fellowship, an NSF CAREER award, recognition as a top young investigator from the MIT Technology Review magazine, a “Brilliant 10” designation from Popular Science, and a 2002 inclusion from Technology Review on annual list of 35 innovators under the age of 35, just to keep things interesting (and lauded).
For now, Kavraki continues to teach at Rice University, with her own Kavraki Lab bent on researching the two prongs of her scientific interests: robotics and bioinformatics and biomedicine. By all accounts, Kavraki’s brilliance in the lab translates to the classroom, as she is a recipient of Rice’s own Duncan Award for excellence in research and teaching. Kavraki’s dedication to the advancement of not only her research, but her students and science in general, is clear enough already, though beautifully minimized with one line on her Rice website linking out to still more accomplishments and awards, those of others dear to her, reading simply, “I am most proud of the accomplishments and awards of my students.”